Table Of Content
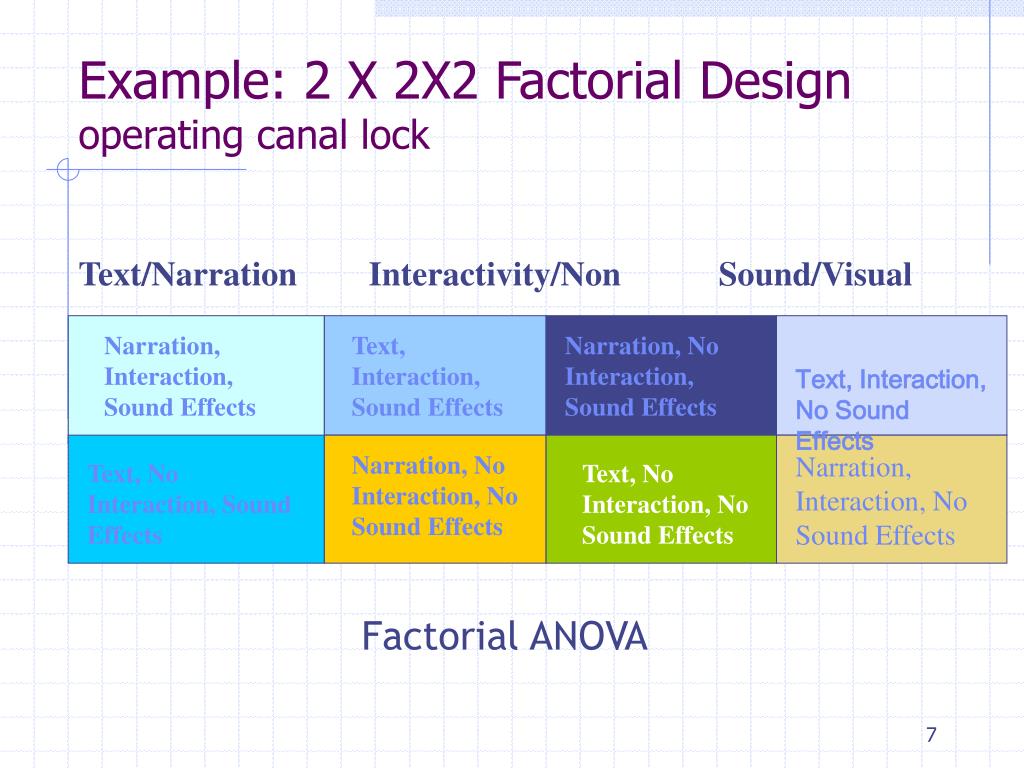
These independent variables are good examples of variables that are truly independent from one another. For example, shoes with a 1 inch sole will always add 1 inch to a person’s height. This will be true no matter whether they wear a hat or not, and no matter how tall the hat is.
How Factorial Survey Analysis Improves Our Understanding of Employer Preferences
In the remainder of this section, we will focus on between-subjects factorial designs only. Also, regardless of the design, the actual assignment of participants to conditions is typically done randomly. Thus far we have seen that factorial experiments can include manipulated independent variables or a combination of manipulated and non-manipulated independent variables. But factorial designs can also include only non-manipulated independent variables, in which case they are no longer experiments but are instead non-experimental in nature.
Visualizing Main Effects & Interaction Effects
Some were negative, health-related words (e.g., tumor, coronary), and others were not health related (e.g., election, geometry). The non-manipulated independent variable was whether participants were high or low in hypochondriasis (excessive concern with ordinary bodily symptoms). Results from this study suggested that participants high in hypochondriasis were better than those low in hypochondriasis at recalling the health-related words, but that they were no better at recalling the non-health-related words. In many factorial designs, one of the independent variables is a non-manipulated independent variable.
MER as Mixed-Factorial ANOVA with Multiple Crossed Factors
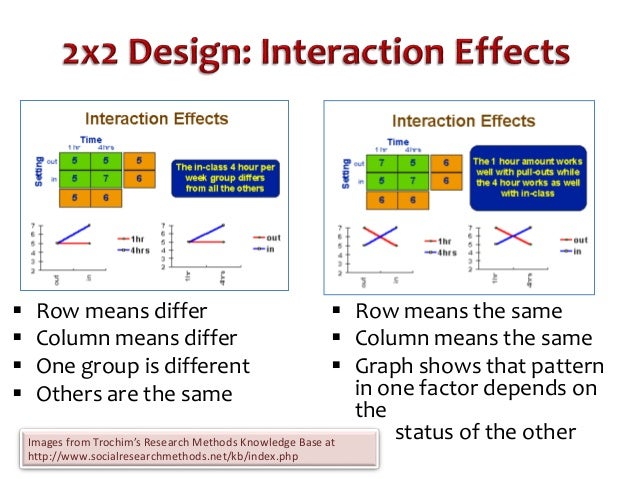
In this case, the error would be the interaction between replicates and unconfounded treatments. This RCBD framework is a foundational structure that we use again and again in experimental design. For this mixed factorial ANOVA, we have two factors that vary withinsubjects, Condition and Time, and we have one factor that varies betweensubjects, Age Group. As before, we can directly code this into ouranalysis of variance using the aov() function or using the ezANOVA()function from the “ez” package. The presence of an interaction, particularly a strong interaction, can sometimes make it challenging to interpet main effects. For example, take a look at Figure 5.14, which indicates a very strong interaction.
MER as One-Way Repeated Measures ANOVA
The question arises whether researchers should plan all these decisions beforehand, or whether they can make them during, and depending on the course of, the research process. On the one hand, a researcher should decide beforehand which research components to include in the design, such that the conclusion that will be drawn will be robust. On the other hand, developments during research execution will sometimes prompt the researcher to decide to add additional components. When one is able to plan for emergence, one should not refrain from doing so.
Mixed Level Designs
Factorial designs require the experimenter to manipulate at least two independent variables. Imagine you are trying to figure out which of two light switches turns on a light. The dependent variable is the light (we measure whether it is on or off).
Analyzing factorial survey data with structural equation models
As we will see, interactions are often among the most interesting results in empirical research. Recall that in a between-subjects single factor design, each participant is tested in only one condition. In principle, factorial designs can include any number of independent variables with any number of levels. For example, an experiment could include the type of psychotherapy (cognitive vs. behavioral), the length of the psychotherapy (2 weeks vs. 2 months), and the sex of the psychotherapist (female vs. male). This would be a 2 x 2 x 2 factorial design and would have eight conditions.
(The y-axis is always reserved for the dependent variable.) Figure 8.3 shows results for two hypothetical factorial experiments. Time of day (day vs. night) is represented by different locations on the x-axis, and cell phone use (no vs. yes) is represented by different-coloured bars. This variable, psychotherapy length, is represented along the x-axis, and the other variable (psychotherapy type) is represented by differently formatted lines. This is a line graph rather than a bar graph because the variable on the x-axis is quantitative with a small number of distinct levels. Line graphs are also appropriate when representing measurements made over a time interval (also referred to as time series information) on the x-axis. It is also possible to manipulate one independent variable between subjects and another within subjects.
Nanotechnology based blended chitosan-pectin hybrid for safe and efficient consolidative antiemetic and neuro ... - ScienceDirect.com
Nanotechnology based blended chitosan-pectin hybrid for safe and efficient consolidative antiemetic and neuro ....
Posted: Fri, 27 Nov 2020 13:53:22 GMT [source]
Simultaneity indicates whether data collection is done concurrent or sequentially. Dependence indicates whether the implementation of one component depends upon the results of data analysis of the other component. As we will see in the example case studies, a concurrent design could include dependent data analysis, and a sequential design could include independent data analysis. It is conceivable that one simultaneously conducts interviews and collects questionnaire data (concurrent), while allowing the analysis focus of the interviews to depend on what emerges from the survey data (dependence).
So people who are high in extraversion might be high or low in conscientiousness, and people who like reflective and complex music might or might not also like intense and rebellious music. The second point is that factor analysis reveals only the underlying structure of the variables. It is up to researchers to interpret and label the factors and to explain the origin of that particular factor structure. For example, one reason that extraversion and the other Big Five operate as separate factors is that they appear to be controlled by different genes [PDMM08].
She conducted an experiment in which the independent variable was whether participants were tested in a room with no odor or in one scented with lemon, lavender, or dimethyl sulfide (which has a cabbage-like smell). Although she was primarily interested in how the odors affected people’s creativity, she was also curious about how they affected people’s moods and perceived health—and it was a simple enough matter to measure these dependent variables too. Although she found that creativity was unaffected by the ambient odor, she found that people’s moods were lower in the dimethyl sulfide condition, and that their perceived health was greater in the lemon condition. The effect of one independent variable can depend on the level of the other in several different ways. This is like the hypothetical driving example where there was a stronger effect of using a cell phone at night than during the day.
Effective Removal of Levofloxacin from Pharmaceutical Wastewater Using Synthesized Zinc Oxid, Graphen Oxid ... - Nature.com
Effective Removal of Levofloxacin from Pharmaceutical Wastewater Using Synthesized Zinc Oxid, Graphen Oxid ....
Posted: Fri, 03 Apr 2020 07:00:00 GMT [source]
Now we assign the treatment combinations to the blocks, where the pairs represent the levels of factors A and B. In our example, participants are nested within age-group, becauseeach person is represented at only one level of that factor (i.e., youare only a younger adult or older adult). However, Condition is acrossed factor, because each person is represented at all levels ofCondition (i.e., each person was measured in all three conditions). For the examples we will walk through, we will ignore differentvariables at different times. As these researchers expected, participants who were lower in SES tended to give away more of their points than participants who were higher in SES. This is consistent with the idea that being lower in SES causes people to be more generous.
The research then also measure participants’ willingness to have unprotected sexual intercourse. This study can be conceptualized as a 2 x 2 factorial design with mood (positive vs. negative) and self-esteem (high vs. low) as between-subjects factors. This design can be represented in a factorial design table and the results in a bar graph of the sort we have already seen.
You can look at the red bars first and see that the red bar for no-shoes is slightly smaller than the red bar for shoes. The green bar for no-shoes is slightly smaller than the green bar for shoes. Except in this case, we find the average heights in the no hat vs. hat conditions by averaging over the shoe variable. To find the main effect of the shoes manipulation we want to find the mean height in the no shoes condition, and compare it to the mean height of the shoes condition. To do this, we collapse, or average over the observations in the hat conditions.
No comments:
Post a Comment